Baker Tilly’s Insights on How To Deliver Business Value From AI
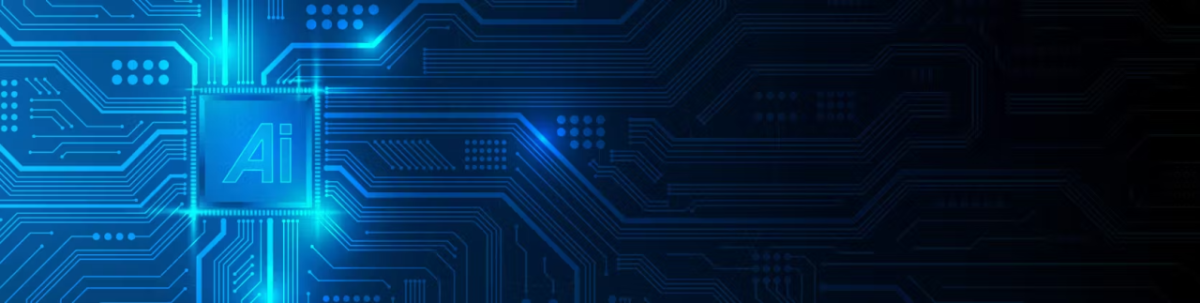
Authored by Thomas M. Puch, Nasir Mahmood, Jessica Drexler
Many organizations are now considering how to harness the potential of AI to drive business transformation and improve operational efficiency. A strategic approach to implementing artificial intelligence (AI) can help companies move from exploratory discussions to impactful solutions.
One promising approach lies in automated decision-making, where companies use AI to drive business value creation. Implementing an automated decision-making application starts with identifying specific business workflows or use cases, identifying upstream dependencies and decomposing decisions down to their atomic level. This initial analysis serves as the scaffolding to identify where appropriate AI, machine learning, statistical or automation technologies can be used.
Workflows involving high-volume decisions or unstructured data such as contracts, invoices, memos, monthly statements, repair notes or legacy operational reports are ideal candidates for automation. Companies often apply these solutions to workflows like reconciliation processes, audits, compliance reviews, revenue recognition and fraud screening/integrity validation.
Starting with AI in automated decision-making often involves the following considerations:
- Process identification: Determining which specific processes or functions are suitable for automation.
- Business value and impact: Defining ways to evaluate and measure the solution to ensure it delivers clear benefits.
- Team composition: Selecting the right team to ensure successful implementation.
- Technical feasibility: Ensuring the solution meets technical requirements, including data security and integration.
- Data readiness/data governance: Confirming that sufficient, accurate and reliable data is available.
- Data management: Identifying opportunities to centralize data.
To maximize the value of your AI solution, prioritize these four key elements:
1. Team composition
Assembling a cross-functional team is key for a successful AI project. The team needs an executive sponsor to lead the initiative, define its goals and allocate necessary resources. The team should also include individuals who have the “know how,” which is the knowledge and skills to describe both a broad understanding of the process and deep knowledge of specific decisions.
These individuals also need to be able to explain quality standards and the evaluation mechanisms. This might include a manager who can outline the overall workflow, as well as subject matter experts who understand the finer details of specific functional areas.
Additionally, technical individuals fluent in system design, AI and machine learning, source system data sources and change management are essential. By bringing together individuals with diverse skills and knowledge, the team will be well-prepared to deliver an effective AI solution.
2. Decision management
Decision management is a framework that, when used properly, significantly increases the likelihood of achieving tangible business outcomes with AI. In fact, if Benjamin Franklin were alive today, he might have said, "An ounce of decision management is worth a hundred pounds of cure."
Decision management is a methodology that, when used correctly, significantly increases the likelihood of your AI solution delivering tangible business value. For those interested in exploring this topic further, James Taylor’s Digital Decisioning: Using Decision Management to Deliver Business Impact from AI [1] offers deeper insights.
Decision management methodology
The first step in using decision management involves collaboratively mapping out the current or ideal logical process flow. This should be done with input from business users who understand the end-to-end workflow, as well as team members familiar with specific steps.
From here, the team should iteratively review the initial logical flow and decompose each decision to its atomic level. This step requires time and care to uncover 'hidden' decisions embedded within the process but not immediately visible. Identifying decisions at the atomic level is essential for ensuring explainability, transparency and measuring data bias.
Next, the team should identify internal and external information sources. These will likely include structured and unstructured data, along with any upstream analytical insights that inform decision-making. It is helpful to involve individuals who can explain the decision-making process and its evaluation from a non-technical, business perspective to ensure a complete understanding.
For each decision, consider factors such as frequency, volume and the required time horizon once all data is available. Define valid responses, acceptable response ranges and the characteristics of a "good" response. This assessment should also assess the value of both "good" and "bad" decisions, along with the time to value (TTV) –the duration it takes to realize the decision’s value. Short TTV decisions allow for quicker feedback, whereas longer TTV decisions often take weeks or even months to evaluate.
The outputs of decision management include a diagram map and a decision inventory document. The diagram map visually breaks down all decisions made in the process, highlighting dependencies such as information sources, know-how and upstream analytics. Meanwhile, the decision inventory lists each decision’s frequency, key performance indicator (KPI), volume, time horizon and acceptable responses.
3. Data management
During exploratory data assessments, it's common to encounter gaps and limitations in both internal and external datasets. Nearly all organizations face similar challenges, the key is to devise strategies to address these gaps.
Many business leaders may not be aware of the role of AI and machine learning can play a significant role in bridging these gaps by standardizing, imputing, inferring, and classifying data to enhance data quality for subsequent AI initiatives. As a recent Gartner presentation [2] suggests, "Not having AI-ready data is no longer a 'showstopper.'" AI and machine learning should be an integral part of preparing data for AI initiatives.
Data management for AI ready data
Implementing automated decision-making applications requires establishing shared data stores to organize and secure data with proper security classifications and access controls. These shared data stores will help safeguard sensitive information while facilitating authorized data access.
Ongoing monitoring for data drift and data bias is essential to ensure the fairness and reliability of the AI solution. Tracking changes in the statistical properties of input data and responses over time ensures that applications are accurate and reliable.
4. Organizational readiness
Integrating organizational change management (OCM) is essential for successfully adopting and embedding AI solutions. Identifying training gaps and upskilling opportunities is a crucial step to maximize ROI. Without adequate preparation, new technologies fail to deliver expected results because the workforce may default to familiar methods, limiting the impact of new tools. Providing the necessary training and support empowers the team to fully leverage the technology and tools effectively, driving meaningful and lasting transformation.
The next crucial step is ensuring that OCM proactively addresses organizational readiness and engages stakeholders to ensure a smooth transition to the new AI solution. This includes assessing readiness for change, resource capacity, developing communication and mitigating potential risks and barriers to utilization and adoption.
Early understanding of people-related impacts resulting from processes and technology decisions will enable the organization to properly prepare users for the changes ahead, building the commitment and desire needed to maximize the benefits of AI and minimize resistance, ultimately driving business value and long-term success.
Reaching the go-live stage is just one component of the journey. Beyond supporting the transition to an automated decision-making application, organizational readiness can further work to instill ownership and accountability for the successful sustainment of the transformation. By beginning with the end in mind, leaders can build a culture where people are enabled to support the organization’s goals and drive growth through the application of AI within its business systems and operating models.
How we can help
Baker Tilly’s approach focuses on identifying high impact use cases and aligning AI initiatives with your strategic goals. By focusing on actual business decision-making, we can help your organization streamline processes, enhance accuracy and accelerate realizing business value from AI.
Ready to take the next step? Contact a Baker Tilly specialist to schedule an AI discovery workshop.