Booz Allen Data Scientists Challenge the Global Data Science Community to Change the World
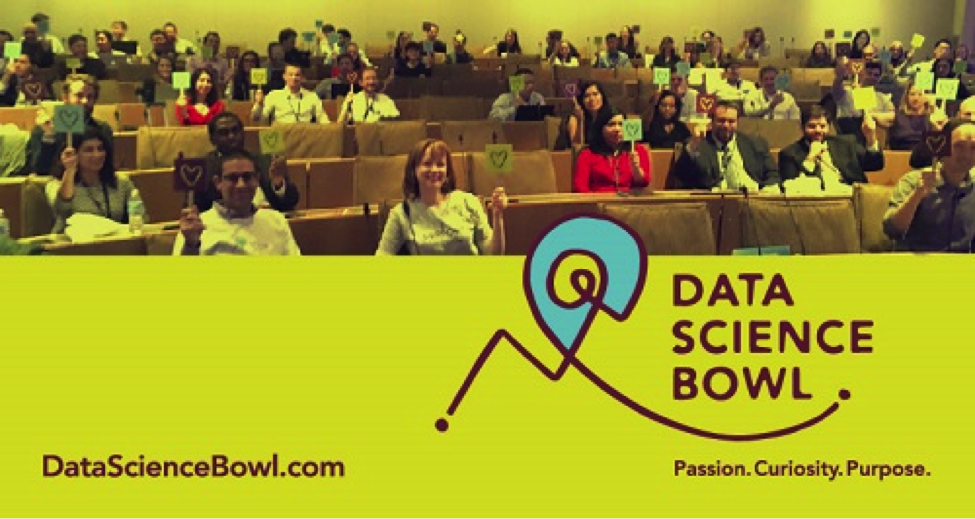
Last week, Booz Allen Hamilton and Kaggle announced the kick-off of the second annual Data Science Bowl. This year’s challenge calls on the global data science community to create a set of steps, or algorithms, to help transform diagnosis of heart disease. By putting data science to work in the cardiology field, data scientists can empower doctors to help people live longer and spend more time with those that they love.
Booz Allen data scientists Alex Cosmas and Michael Abramovich authored this blog post, calling on the global community of data scientists to do their part to change the world:
So you can predict the future. Big Deal. Now change it.
It’s time for us to push the envelope as a data science community. We’ve proven our ability to find the most obscure of facts (e.g., humans share 50% of their DNA with bananas). We’ve uncovered patterns in untamable datasets that lead to ground-breaking insights. We’ve even learned how to predict the future.
But that is no longer enough. Our audiences want to know why things are happening, and more notably, how to change the futures we predict. They want the identification of root causes, typically the product of human reasoning and experience. Influence requires intervention–and intervention requires an understanding of the rules of a system. That requires causal–rather than simply observational–inference.
While we always heed the old adage “correlation does not imply causation,” many data scientists lack the understanding of the specific conditions under which it is acceptable to derive causal interpretations from correlations in observational data.
How can we isolate the effect of a drug treatment, a marketing campaign, or a policy from observational data? We don’t always have the opportunity to run a prospective trial with randomized assignment–the gold standard for causal interpretation. Sometimes all we have to work with is what’s already happened. The Neyman-Rubin causal model provides us a baseline framework for causal inference, but given the unavoidable imperfections of experimental design and data collection, we must address the prevalence of covariates and confounders. We can treat covariates through statistical matching methods, like propensity score matching or Mahalanobis distance computation. Furthermore, Judea Pearl’s do-calculus enables the treatment of confounders through statistical “intervention.”
The million-dollar question is: can we machine-learn causality? Getting causal is no small feat. But it’s not insurmountable. Today, we are able to employ heuristics alongside machine-learned models to eliminate causation. For example:
- Causes and effects must demonstrate mutual information (or Pearson’s correlation in linear models) i.e. observation of random variable X (cause) must reduce the uncertainty of Y (effect)
- Temporalization: causes must occur before effects. This is obvious, but incredibly powerful in eliminating causality. Simultaneous observation of random variables (e.g., rainfall and a wet lawn) is often simply a limitation in the fidelity of data collection.
- Networks of cause and effect: multiple effects of the same cause cannot demonstrate partial information flow. For example, we cannot observe multiple lawns–some wet and some not–and conclude that rainfall is the exclusive root cause. Instead, there is either an additional (perhaps latent) cause or rainfall is not the cause.
If we don’t have mutual information, proper temporalization, and a single cause, we know we can’t approach causality. But, as we recall from standardized exams, the process of elimination often leads to the correct answer.
We, as data scientists, will continue to be called upon to solve the toughest problems. We cannot get away with relying upon machine-learning to do machine-thinking. To earn our place in the science community, we must become stewards of the scientific method–of theory formulation and scientific reasoning. In other words, we must become truth-seekers, not simply fact-seekers.
To learn more about the Data Science Bowl, and to register to compete, visit: http://www.datasciencebowl.com.